Learning Approach for Segmentation
Multi-organ segmentation
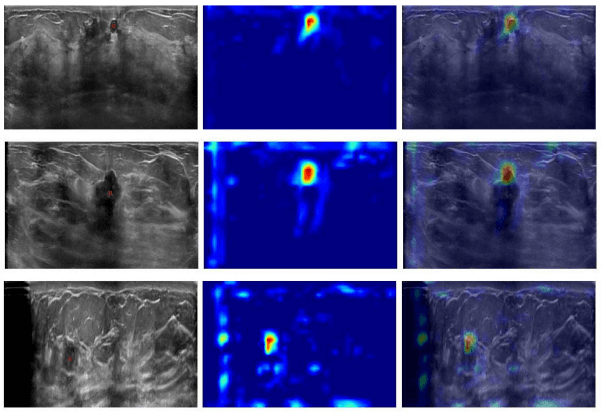
In order to detect lesions on medical images, deep learning models commonly require information about the size of the lesion, either through a bounding box or through the pixel-/voxel-wise annotation of the lesion, which is in turn extremely expensive to produce in most cases.
In this paper, we aim at demonstrating that by having a single central point per lesion as ground truth for 3D ultrasounds, accurate deep learning models for lesion detection can be trained, leading to precise visualizations using Grad-CAM. From a set of breast ultrasound volumes, healthy and diseased patches were used to train a deep convolutional neural network. On the one hand, each diseased patch contained in its central area a lesion’s center annotated by experts. On the other hand, healthy patches were extracted from random regions of ultrasounds taken from healthy patients.In order to detect lesions on medical images, deep learning models commonly require information about the size of the lesion, either through a bounding box or through the pixel-/voxel-wise annotation of the lesion, which is in turn extremely expensive to produce in most cases.
In this paper, we aim at demonstrating that by having a single central point per lesion as ground truth for 3D ultrasounds, accurate deep learning models for lesion detection can be trained, leading to precise visualizations using Grad-CAM. From a set of breast ultrasound volumes, healthy and diseased patches were used to train a deep convolutional neural network. On the one hand, each diseased patch contained in its central area a lesion’s center annotated by experts. On the other hand, healthy patches were extracted from random regions of ultrasounds taken from healthy patients.