HookFormer: Revolutionizing Glacier Calving Front Detection with Advanced Transformer Networks
In the rapidly evolving field of remote sensing and environmental monitoring, the need for accurate, efficient, and automated analysis of satellite imagery has never been more critical. This is especially true in the context of observing glacier dynamics, where changes in calving fronts serve as crucial indicators of climate change impacts. The article in focus presents a groundbreaking method named HookFormer, a novel deep learning architecture designed to address the challenges of glacier calving front detection in synthetic aperture radar (SAR) images.
Introduction to Glacier Monitoring Challenges
Glacier calving fronts, the boundary at which glaciers release icebergs into the ocean, are key to understanding glacier dynamics and their contribution to sea level rise. Traditionally, monitoring these fronts required manual delineation in remote sensing imagery—a labor-intensive and costly process susceptible to human error. The advent of deep learning has revolutionized this task, offering automated solutions that promise speed, accuracy, and reduced costs. Yet, the field has faced hurdles, primarily due to the scarcity of diverse training images and the subtle geometric changes in glacier fronts, which complicate the segmentation task.
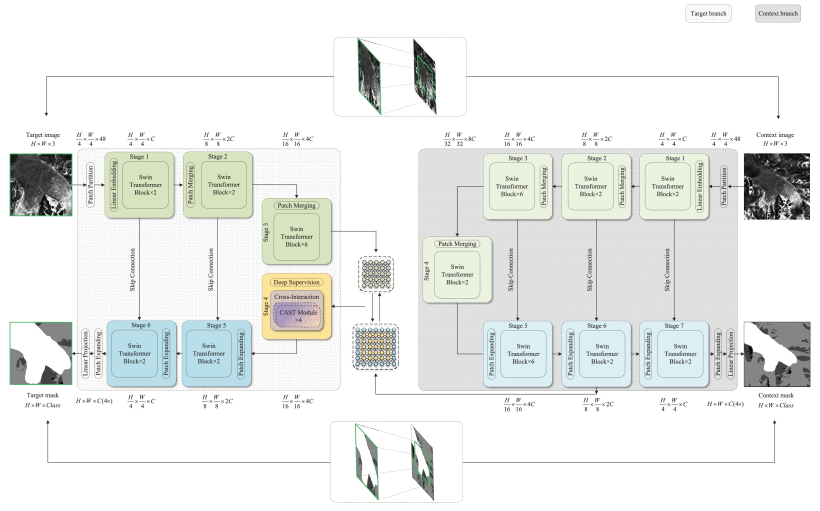
Figure 1: Overview of our HookFormer approach. A target branch and a context branch are involved in a unified end-to-end ViT framework, where the target input is center-cropped from the context input but equipped with a higher image resolution, the context input has more surroundings and is downsampled to match the size of the target input leading to a lower resolution. The two branches are connected by the proposed cross-attention and cross-interaction modules, supervised and optimized by the corresponding ground truth.
The Novelty of HookFormer
HookFormer emerges as a solution, harnessing the power of transformer architectures—a technology that has shown remarkable success across various domains of computer vision. The approach introduces an innovative method for detecting glacier calving fronts by leveraging the strengths of synthetic aperture radar (SAR) imagery. This method ingeniously combines high-resolution target inputs with low-resolution context inputs within a unified transformer architecture, ensuring a comprehensive analysis that integrates both detailed local views and broader contextual information. By center-cropping the target input from the original context input and downsampling the latter to match the target’s size, HookFormer maintains the contextual integrity of the data while focusing on specific areas of interest. This dual-input strategy enhances the model’s ability to discern critical features in the glacier fronts by providing a more holistic understanding of the surrounding environment.
At the core of HookFormer’s architecture are the Swin-Transformer blocks, which form the backbone of both the high-resolution and low-resolution branches. These blocks utilize a hierarchical structure based on alternately shifted-window-based self-attention mechanisms, achieving state-of-the-art performance in various vision tasks. This design enables the model to effectively capture both global and local dependencies within the imagery, a critical aspect for accurately identifying glacier calving fronts. The Swin-Transformer blocks are characterized by their efficient handling of multihead self-attention, LayerNorm (LN) layers, and multilayer perceptron (MLP) with Gaussian error linear unit (GELU) nonlinearity, ensuring robust feature extraction and processing.
To further enhance the model’s capabilities, HookFormer incorporates a Cross-Attention Swin-Transformer (CAST) module, designed to facilitate the interaction between the context and target branches. This module employs a novel cross-attention mechanism that allows for the dynamic integration of coarse-grained context and fine-grained target feature representations. Through a series of two cross-attention transformer blocks, the CAST module effectively models the dependencies between different levels of detail within the imagery, enabling the model to adjust its focus dynamically and accurately delineate glacier calving fronts.
Moreover, the Cross-Interaction module plays a pivotal role in optimizing the fusion of multiresolution features, addressing a significant challenge in multiscale CNNs and Vision Transformers (ViTs). By alternately changing the query set and the key-value sets within the CAST module, this module ensures a comprehensive and effective integration of information across the target and context branches. The Cross-Interaction module’s design not only enhances the model’s accuracy in segmenting glacier fronts but also improves the overall efficiency of the feature fusion process, demonstrating the advanced capabilities of HookFormer in processing complex SAR imagery for environmental monitoring applications.
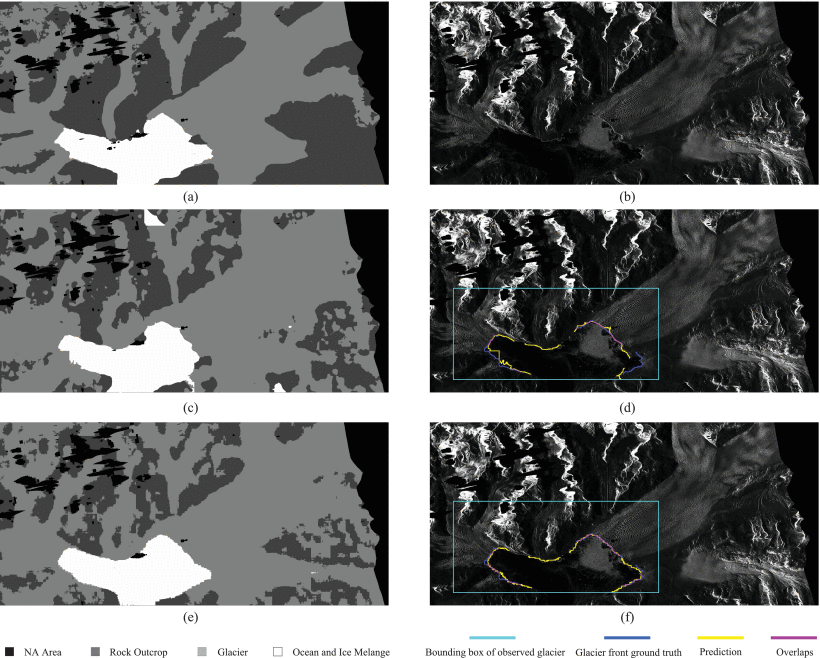
Figure 2: Qualitative comparison using the example of an SAR image of the Columbia Glacier (b) taken on the 11th of February 2016 by the TanDEM-X (TDX) satellite. (a) Annotated segmentation ground truth of (b), where white represents the ocean, light gray the ice, dark gray the rock outcrop, and black a “no data available” region. (c) and (e) Predicted segmentation maps from AMD-HookNet and our HookFormer, respectively. (d) and (f) Corresponding glacier calving fronts delineated by postprocessing of the CaFFe benchmark, where blue, yellow, and purple indicate the glacier front ground truth, the detected glacier front, and the overlap between the prediction and the glacier front ground truth, respectively.
Empirical Validation
The comprehensive analysis of HookFormer’s performance on the CaFFe dataset showcases its superiority over existing state-of-the-art methods for glacier calving front detection, including AMD-HookNet, Swin-Unet, HookNet, and nnU-Net OCFD. HookFormer excels in all metrics except precision, with notable improvements in Intersection over Union (IoU) and Mean Distance Error (MDE), indicating its robustness and precision in delineating glacier calving fronts. This performance leap underscores the model’s innovative approach to integrating high-resolution target inputs with low-resolution context inputs, optimizing the detection process through advanced transformer architectures.
The segmentation performance comparison between HookFormer and AMD-HookNet highlights HookFormer’s superior accuracy in classifying glacier and ocean classes, which is pivotal for accurately delineating calving fronts. This improvement is attributed to HookFormer’s ability to effectively harness global and local information, enhancing the model’s performance in both glacier segmentation and calving front delineation. Qualitative visualizations further illustrate HookFormer’s precision in mapping glacier calving fronts, showcasing its potential to significantly advance the field of remote sensing for glacier monitoring.
The detailed performance breakdown across different glaciers and seasons reveals HookFormer’s effectiveness in various conditions, significantly reducing the MDE across all scenarios compared to AMD-HookNet. This indicates HookFormer’s adaptability and reliability in accurately identifying glacier calving fronts, even in challenging winter conditions where distinguishing between sea ice and glacier ice becomes more complex. The model’s performance is consistently superior, demonstrating its capacity to provide more accurate and reliable data for studying glacier dynamics and their impact on sea level rise.
The ablation studies emphasize the critical components contributing to HookFormer’s success, including deep supervision, the CAST module, and the cross-interaction module. These components are pivotal in enhancing the model’s efficiency and accuracy, highlighting the innovative approach of integrating transformer architectures for environmental monitoring tasks. HookFormer’s significant performance gains, coupled with a reduction in model parameters and computational complexity, mark a substantial advancement in the application of deep learning technologies for the precise and efficient monitoring of glacier calving fronts.
Conclusion and Future Directions
HookFormer represents a significant leap forward in the automated analysis of glacier dynamics, offering a robust, efficient, and highly accurate method for monitoring calving fronts. Its success opens avenues for further research into transformer-based architectures for environmental monitoring and beyond. Future work may explore the integration of additional modalities, such as optical imagery, and the application of HookFormer’s principles to other domains requiring precise segmentation capabilities.
This study not only contributes to the field of remote sensing and environmental science but also showcases the transformative potential of deep learning in tackling some of the most pressing challenges of our time.
If you liked this essay, you can have a look at my YouTube Channel. This article is released under the Creative Commons 4.0 Attribution License and was created with the aid of GPT4.
Reference
Wu, Fei, Nora Gourmelon, Thorsten Seehaus, Jianlin Zhang, Matthias Braun, Andreas Maier, and Vincent Christlein. “Contextual HookFormer for Glacier Calving Front Segmentation.” IEEE Transactions on Geoscience and Remote Sensing (2024). https://ieeexplore.ieee.org/document/10440599?source=authoralert