Invited Talk by Markus Haltmeier, Univ. Insbruck, Jan 13th 2021, 14h CET
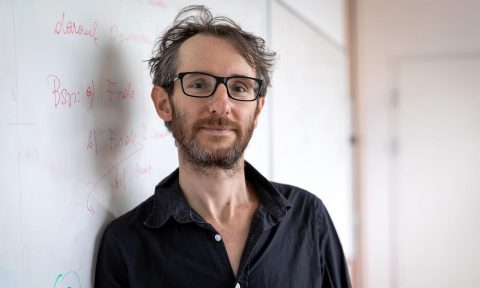
It is a great pleasure to welcome Prof. Dr. Markus Haltmeier at our lab next week!
Title: Learned Analysis and Synthesis Regularisation of Inverse Problems
Date: Jan 13th 2021, 14h CET
Location: https://fau.zoom.us/j/92450392476?pwd=WnVKSWJ4V3pMck1CZUlvVVREelYzZz09
Abstract: Inverse problems consist of finding accurate approximations for the unknown. Unknown x from noisy data y = A (x) + b, where A is the so-called forward operator, b represents the noise (data perturbation) and y are given noisy data. The characteristic property of inverse problems is their ill-posedness, which means that A(x) = y has no unique solution or the solution depends unstably on the given data. To obtain stable and accurate solutions, regularisation methods incorporate additional available information about the unknown and the noise. In this talk, we review classical frame-based analysis and synthesis regularisation. We then present recent extensions that use neural networks as nonlinear synthesis and analysis operators. A mathematical analysis is given, possible training strategies are discussed and connections to related work are presented. This talk is based on:
[1] H. Li, J. Schwab, S. Antholzer, M. Haltmeier, M. NETT: Solving inverse problems with deep neural networks. Inverse Problems 36, 2020.
[2] D. Obmann, L. Nguyen, J. Schwab, M. Haltmeier. Sparse l^q-regularization of inverse problems with deep learning, arXiv:1908.03006 [math.NA], 2020.
[3] D. Obmann, J. Schwab, M. Haltmeier. Deep synthesis regularization of inverse problems, Inverse Problems 37, 2021.
Short Bio: Markus Haltmeier received his Ph.D. in mathematics from the University of Innsbruck, Tyrol, Austria, in 2007 for his work on computed tomography. He then worked as a researcher at the University of Innsbruck, the University of Vienna, Austria, and the Max Planck Institute for Biophysical Chemistry in Göttingen, Germany, on various aspects of inverse problems. Since 2012, he is a full professor at the Department of Mathematics, University of Innsbruck. His current research interests include inverse problems, regularisation theory, signal and image processing, computed tomography, photoacoustic imaging, and machine learning.