Diffusion weighted echo planar imaging pulse sequences are commonly used for clinical routine Magnetic Resonance Imaging, e.g., for stroke or tumor assessment. These sequences, especially if acquired in a segmented approach, are prone to artefacts such as ghosting, geometric distortions, blurring and mesh artefacts. These can be caused due to patient physiology, hardware imperfections or mismatches between acquired segments. There exists a variety of software-based correction approaches to counter these artefacts which however fail in various scenarios. The goal of this thesis is to evaluate deep learning based correction approaches to improve the image quality compared to conventionally implemented correction approaches. An example for this is shown below.
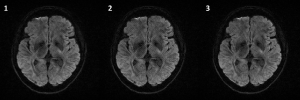
The figure above shows Partial Fourier reconstruction for a readout segmented EPI acquisition (RESOLVE). The Zero filled reconstruction is blurry and the POCS reconstruction suffers from mesh/stripe artefacts. The goal is to train a neural network to perform Partial Fourier reconstruction to overcome the downsides of the conventional approaches.