Index
Transferring of emotional knowledge: from quantitative emotions to qualitative emotions
X-ray image synthesis via an open source framework
Use a new open source framework generating X-ray images for deep learning model training.
Requirement: Python, CT reconstruction
Please attach your CV and transcripts to fuxin.fan@fau.de
Geometric Domain Adaptation for CBCT Segmentation
In this project, we perform a computational domain transfer to introduce cone-beam artifacts to the training data. We evaluate its impact on the results of supervised training for the segmentation of the lungs. For this, already labeled CT volumes are reconstructed to artificial CBCT volumes without a complex deep learning-based method, like introduced by Jia X et al.,5 but rather by computational reconstruction. The purpose is to have a network for stable segmentation on real CBCT volumes. A major advantage of our approach is that the artificial
CBCT volumes can not only be computed easily from thoracic CT volumes but also the pixel-wise segmentation can be re-used without putting in the great effort of labeling. This allows for supervised training.
Realistic Simulation of Collimated X-Ray images for Collimator Edge Segmentation using Deep Learning
Collimator detection in X-ray systems has long posed a challenge, particularly when information about the detector’s position relative to the source is either unreliable or completely unavailable. In this paper [1], we introduce a physically motivated image processing pipeline designed to simulate the intricate characteristics of collimator shadows in X-ray images. The primary objective of this pipeline is to address the scarcity of training data for deep neural networks, which are increasingly promising for collimator detection. By applying the pipeline to deep networks initially limited by small datasets, our approach equips them with the necessary information to learn and generalize effectively.
Our pipeline is a comprehensive solution that leverages several key components to
generate realistic collimator images. Employing randomized labels to describe collimator shapes and their respective locations ensures diversity and representativeness. In addition, we integrate a convolution kernel based scattered radiation simulation mechanism, which is a crucial factor in real-world X-ray imaging. To complete the simulation process, we introduce Poisson noise to replicate the inherent characteristics of collimator shadows in X-ray images.
Comparing the simulated data with real collimator shadows demonstrates the authenticity of our approach and its potential to bridge the gap between synthetic and real-world data. Moreover, incorporating simulated data into our deep learning framework not only serves as a valid substitute for real collimators but also significantly improves generalization in real-world applications, holding great promise for the field of collimator detection.
This work was presented at the DALI workshop at the MICCAI conference in Vancouver, Canada and was published in the proceedings:
1. El-Zein B, Eckert D, Weber T, Rohleder M, Ritschl L, Kappler S et al. A Realistic Collimated
X-Ray Image Simulation Pipeline. Data Augmentation, Labelling, and Imperfections – Third
MICCAIWorkshop, DALI 2023, Held in Conjunction with MICCAI 2023, Vancouver, October
12, 2023, Proceedings. Springer Nature. 2023, (in press)
Automatic recognition of bavarian dialects
BT-MP-BavarianDialect_IdeaLet Transformer Decoder for Text Spotting on Historical Maps
HistoricalMapTextSpotting_FlorianKordonBrain Tumour Segmentation Focused on Complex Sub-regions
Deep Reinforcement Learning Based Emergency Department Optimization
Investigating the Possibilities of CT Reconstruction using Fourier Neural Operator
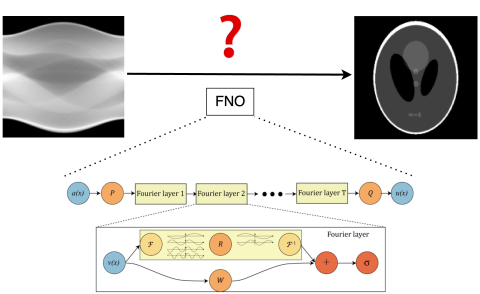
Introduction:
The aim of this project is to explore the potential of using Fourier Neural Operators (FNO) for Computed Tomography(CT) reconstruction tasks. FNO is a novel deep learning framework designed to approximate the solution of Partial Differential Equations (PDEs) by learning continuous operators using a Fourier basis. On the other hand, CT reconstruction is an important process where cross-sectional images of the objects are generated from projections obtained from X-ray scans.
CT reconstruction fundamentally represents an inverse problem, with the objective of recovering the original image from the projection data. This process can be mathematically construed through the lens of a PDE, wherein the Radon transform and its inversion emerge as central figures. By conceptualizing CT reconstruction as a PDE-solving endeavor, we aim to harness the potential of FNO to devise efficient and accurate reconstruction algorithms.
Requirements:
- Completion of at least one course on Deep Learning is mandatory.
- Proficiency in PyTorch is essential.
- Strong analytical and problem-solving skills.
Prospective candidates are warmly invited to send their CV and transcript to yipeng.sun@fau.de.
References:
- Li, Zongyi, et al. “Fourier neural operator for parametric partial differential equations.” arXiv preprint arXiv:2010.08895 (2020).
- Ongie, Gregory, et al. “Deep learning techniques for inverse problems in imaging.” IEEE Journal on Selected Areas in Information Theory 1.1 (2020): 39-56.
Project SENSATION: Sidewalk Environment Detection System for Assistive NavigaTION
In the project entitled Sidewalk Environment Detection System for Assistive NavigaTION (hereinafter referred to as SENSATION), our research team is meticulously advancing the development of the components of SENSATION. The primary objective of this venture is to enhance the mobility capabilities of blind or visually impaired persons (BVIPs) by ensuring safer and more efficient navigation on pedestrian pathways.
For the implementation phase, a specialized prototype was engineered: a chest-bag equipped with an NVIDIA Jetson Nano, serving as the core computational unit. This device integrates a several sensors including, but not limited to, tactile feedback mechanisms (vibration motors) for direction indication, optical sensors (webcam) for environmental data acquisition, wireless communication modules (Wi-Fi antenna) for internet connectivity, and geospatial positioning units (GPS sensors) for real-time location tracking.
Despite the promising preliminary design of the prototype, several technical challenges remain that demand investigation. These challenges are described as follows:
Sidewalk segmentation for direction estimation
To determine the location of a BVIP on the pedestrian pathway, it is imperative for our algorithms to achieve optimal segmentation of the sidewalk. To facilitate this, we continuously refine our proprietary dataset tailored to sidewalk segmentation. We are also exploring a variety of Deep Learning methodologies to enhance the accuracy of this segmentation. The primary objective in this topic is to refine our sidewalk segmentation pipeline and to comprehensively evaluate its performance using metrics such as Mean Intersection over Union (IOU), and precision metrics for both sidewalks and roads. Additionally, we employ Active Learning techniques to further analyze our dataset, aiming to gain a deeper insight into its characteristics.
Distance estimation for obstacles avoidance
To convey information to a BVIP regarding the presence of an impediment on the pedestrian pathway, obstacles such as bicycles, e-scooters, or automobiles must initially be identified via image segmentation techniques. After this identification, it is crucial to determine the distance from these detected objects. The SENSATION system employs a monocular camera to capture the surrounding environmental details of the pathway. In this domain of research, we are studying various algorithms tailored for depth estimation to determine the proximity to these impediments. The calculated distances are then conveyed to the BVIP through either tactile or auditory feedback mechanisms. A prominent challenge in this work lies in achieving precise distance measurements, particularly given the constraints of solely utilizing information from a monocular camera.
Drift correction to improve orientation of a BVIP
While navigating pedestrian pathways, it is occasionally observed that a BVIP may lose orientation with respect to the sidewalk. Addressing this, it is essential to devise a detection system capable of promptly identifying a BVIP’s deviation from the intended sidewalk. For the detection of such drifts, we employ Deep Learning algorithms that leverage optical flow or depth maps. The primary objective in this topic is to conceptualize and develop a drift correction mechanism utilizing either optical flow or depth maps to enhance a BVIP’s sidewalk orientation.
Environmental information by image captioning
To augment a BVIP’s comprehension of their surrounding environment, descriptive captions derived from environmental observations are beneficial. Examples of such captions include: “Traffic light located on your right,” “Staircase descending with a total of 5 steps,” and “Vehicle parked obstructing the sidewalk.”
In this topic, we are examining Deep Learning algorithms that possess the capacity to generate such descriptive annotations. Concurrently, we are refining our caption generation pipeline to ascertain the spectrum of captions that can be formulated to enhance the mobility and spatial understanding of a BVIP.
If you are interested in one of the above topics, please send your request to: hakan.calim@fau.de
For the development of the solutions, it will be beneficial to have experience with implementing neural networks in python with Pytorch or Tensorflow.