Invited Talk: Dr. Zongwei Zhou (Johns Hopkins University) – Body Maps: Towards 3D Atlas of Human Body, Jan 24th 2025, 15h CET
It’s a great pleasure to welcome Dr. Zongwei Zhou to our lab!
Title: Body Maps: Towards 3D Atlas of Human Body
Date: Jan 24th 2025, 15h CET
Location: https://fau.zoom-x.de/j/61681264798?pwd=FdE0HOa1534biXT8xjp64QG5hXgRbu.1
Abstract:
Cancer, a leading cause of mortality, can be effectively treated if detected in its early stages. However, early detection is challenging for both humans and computers. While AI can identify details beyond human perception, delineate anatomical structures, and localize abnormalities in medical images, the training of these algorithms requires large-scale datasets and comprehensive annotations. Several disciplines, such as natural language processing (e.g., GPTs), representation learning (e.g., MAE), and image segmentation (e.g., SAMs), have witnessed the transformative power of scaling data for AI advancement, but this concept remains relatively underexplored in medical imaging, particularly cancer imaging, due to the inherent challenges in data and annotation curation. This talk seeks to bridge this gap by focusing on datasets, annotations, and algorithms that are integral to the analysis of medical images.
Short Bio:
Zongwei Zhou is an Assistant Research Scientist at Johns Hopkins University. He received his Ph.D. at Arizona State University in 2021. His research focuses on developing novel methods to reduce the annotation efforts for computer-aided detection and diagnosis. Zongwei received the AMIA Doctoral Dissertation Award in 2022, the Elsevier-MedIA Best Paper Award in 2020, and the MICCAI Young Scientist Award in 2019. In addition to seven U.S. patents, Zongwei has published over 30 peer-reviewed journal/conference articles, two of which have been ranked among the most popular articles in IEEE TMI and the highest-cited article in EJNMMI Research. He was named the top 2% of Scientists released by Stanford University in 2022 – 2024.
Paper Link:
https://link.springer.com/chapter/10.1007/978-3-031-72378-0_4
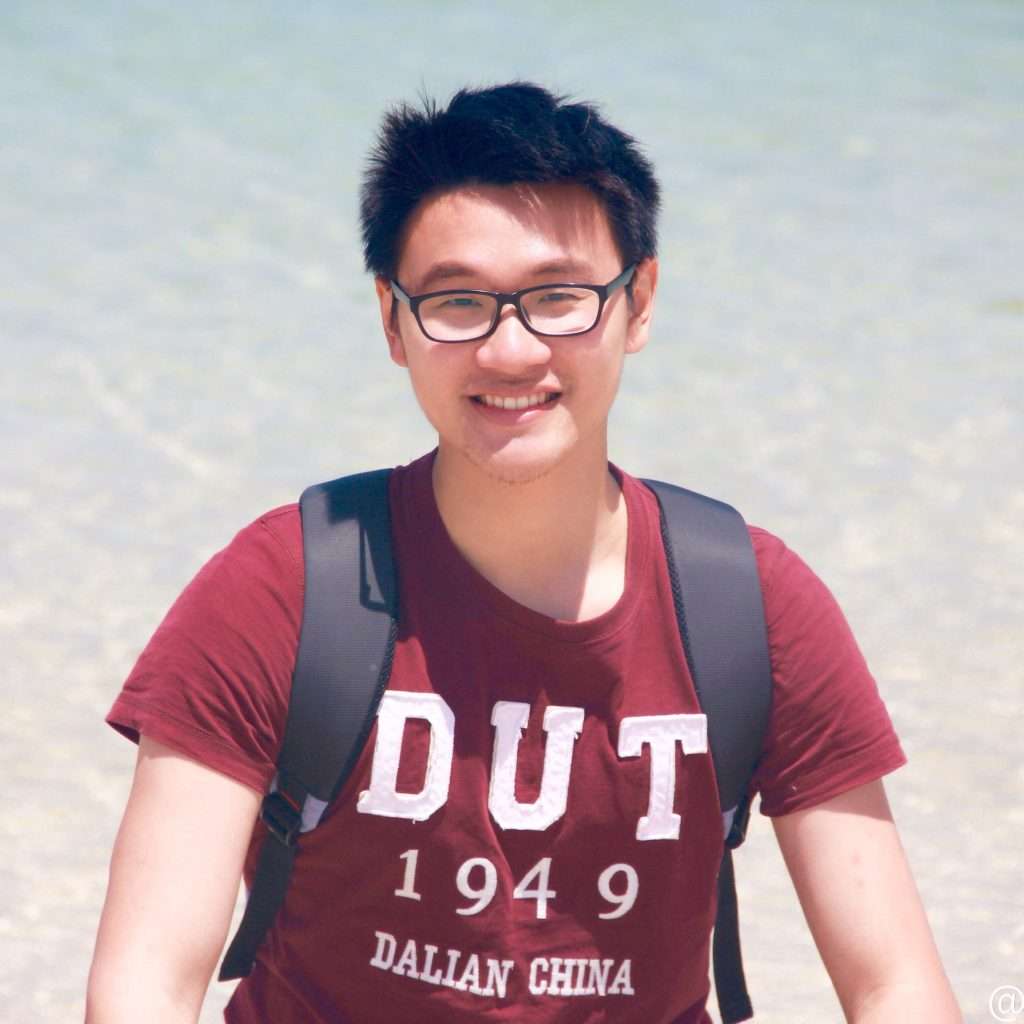