Two Awards at #BVM2021 for the Pattern Recognition Lab @UniFAU, Best Presentation: Katharina Breininger, Best Paper: Tristan Gottschalk Congratulations!
It’s a great pleasure to announce that colleagues of the Pattern Recognition Lab were again successful at the German Conference for Medical Image Processing “BVM 2021”. In this year, our group was able to win two awards at this exciting German conference.
Katarina Breininger won the Best Presentation Award for her Abstract-Submission “Move Over There One-click Deformation Correction for Image Fusion during Endovascular Aortic Repair” which was already awarded the MICCAI Young Scientist Award on MICCAI 2020. Congratulations to Katharina and her Collaborators at Siemens Healhineers!
Paper Abstract: Endovascular aortic repair (EVAR) is an X-ray guided procedure for treating aortic aneurysms with the goal to prevent rupture. During this minimally invasive intervention, stent grafts are inserted into the vasculature to support the diseased vessel wall. By overlaying information from preoperative 3-D imaging onto the intraoperative images, radiation exposure, contrast agent volume, and procedure time can be reduced.
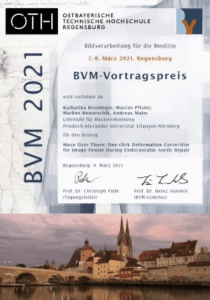
The best scientific contribution this year at BVM was shared and we are glad that one of the Awardees is Tristan Gottschalk for this paper “Learning-based Patch-wise Metal Segmentation with Consistency Check” in which he proposes a cross-projection consistency check to improve the outcomes of metal segmentation for metal artifact reduction in C-arm CT. Also in this case we congratulate Tristan and our colleagues at Siemens Healthineers to this great success!
Paper Abstract: Metal implants that are inserted into the patient’s body during trauma interventions cause heavy artifacts in 3D X-ray acquisitions. Metal Artifact Reduction (MAR) methods, whose first step is always a segmentation of the present metal objects, try to remove these artifacts. Thereby, the segmentation is a crucial task which has strong influence on the MAR’s outcome. This study proposes and evaluates a learning-based patch-wise segmentation network and a newly proposed Consistency Check as post-processing step. The combination of the learned segmentation and Consistency Check reaches a high segmentation performance with an average IoU score of 0.924 on the test set. Furthermore, the Consistency Check proves the ability to significantly reduce false positive segmentations whilst simultaneously ensuring consistent segmentations.
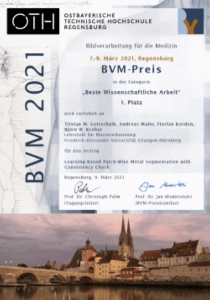